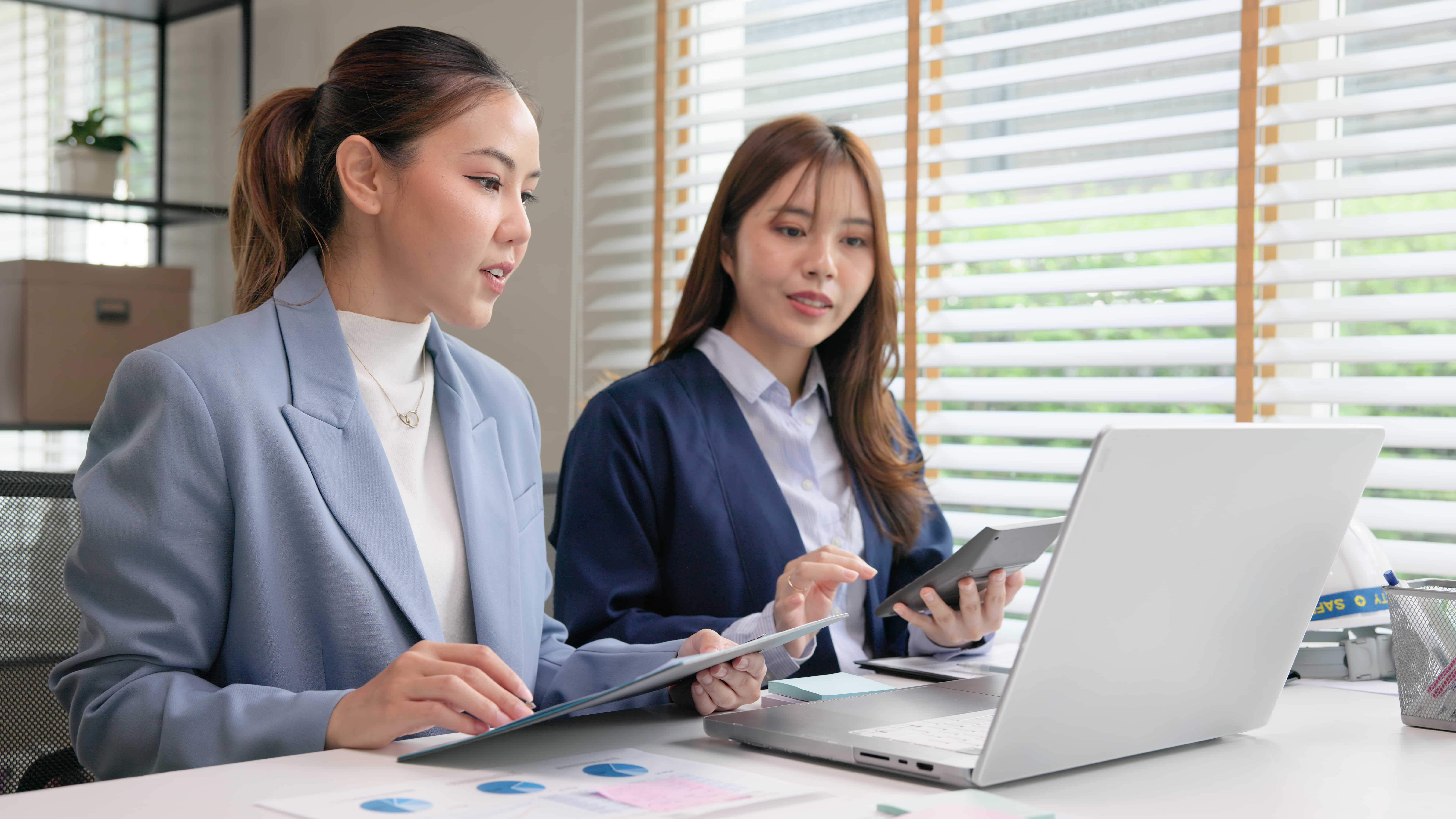
The evolution of artificial intelligence in finance spans five generations, from rules-based automation to agentic AI. Each phase introduced new capabilities—from basic categorization to autonomous decision-making—reshaping how financial teams manage accuracy, speed, and complexity.
Modern finance teams are under pressure to move faster, stay accurate, and remain audit-ready — all while juggling complex systems and limited resources. To meet these demands, many are rethinking their workflows, shifting from manual processes and spreadsheets to smarter, AI-enabled tools tailored for finance.
The evolution of artificial intelligence in finance has been central to this transformation. As noted in McKinsey's The State of AI in 2024 report, 72% of surveyed organizations now use AI regularly in at least one business function — especially in areas like predictive analytics, forecasting, and process automation.
These tools are no longer experimental; they're driving measurable gains in efficiency and performance.
AI’s journey in finance has progressed through multiple generations: each bringing new capabilities, from rule-based logic to machine learning, deep learning, and now agentic systems.
For finance professionals, understanding this evolution is critical to evaluating what’s possible today and what’s coming next.
Let's explore how AI has transformed from simple automation to the new agentic era.
The Evolution of Artificial Intelligence in Finance: 1980s to Today
Over the last four decades, artificial intelligence has evolved through five distinct generations, each introducing new capabilities that have fundamentally changed how finance teams operate.
From static rule-based systems to autonomous agents, every stage has built upon the last, enabling greater speed, intelligence, and adaptability in financial workflows.
Let’s break down each generation to understand how we arrived at the agentic era, and what it means for modern finance teams.
First Generation: Rules-Based AI (1980s-2000s)
The first generation of AI consisted of programmed rules and basic automation, following specific "if-then" sequences.
Real-World Applications:
- Basic transaction categorization
- Simple spreadsheet automation
- Predefined reporting templates
- Automated account reconciliation rules
These systems revolutionized basic bookkeeping but required extensive human oversight and couldn't handle exceptions well.
Forbe’s article “The Truth About Why RPA (Robotic Process Automation) Fail to Scale” indicates that early automation systems were geared toward well-defined data formats, but struggled with unstructured data or process variations.
Explore more on this topic: Modern Accounting Tools: Excel vs RPA vs AI Agents Compared
Second Generation: Machine Learning AI (2010s)
The second generation marked a significant shift in the evolution of artificial intelligence in finance. Instead of relying on fixed rules, machine learning models began identifying patterns in data and improving their performance over time.
These systems enabled more adaptive, data-driven decision-making—especially in areas with large volumes of structured financial data.
Real-World Applications:
- Fraud detection and transaction anomaly alerts
- Intelligent document classification and extraction
- Predictive analytics for cash flow forecasting
- Smart expense categorization based on behavior
- Pattern recognition in complex financial statements
Machine learning made financial systems more proactive, allowing them to detect outliers, flag inconsistencies, and automate insights.
However, these systems were still limited by the quality of input data and lacked transparency, making explainability a growing concern for compliance-heavy environments.
Third Generation: Deep Learning AI (Late 2010s)
Building on machine learning, deep learning introduced neural networks capable of processing massive volumes of complex, high-dimensional data.
These models could detect subtle patterns and relationships in ways that previous generations could not, unlocking a new level of accuracy and automation in financial workflows.
Real-World Applications:
- Natural language processing (NLP) for contracts and financial reports
- Real-time risk assessment models
- Automated audit procedures
- Voice and chat-based virtual finance assistants
- Anomaly detection in transactional data
Deep learning allowed financial teams to move beyond rule-based logic and simple predictions into real-time, intelligent analysis.
This generation of AI enabled greater personalization and automation, but came with increased demands for computational power and faced challenges around data privacy and explainability.
Keep Reading: Agentic AI and the Future of Accounting Workflows
Fourth Generation: Large Language Models (2020s)
Large Language Models (LLMs) marked a major leap in the evolution of artificial intelligence in finance. These models, trained on vast datasets, can understand and generate human-like text, making it possible to interact with systems using natural language instead of rigid inputs.
This generation introduced a more intuitive way for finance professionals to engage with AI.
Real-World Applications:
- Automated financial report generation
- AI-powered customer support and help desks
- Drafting audit narratives and commentary
- Market summaries and real-time financial news analysis
- NLP-based search across financial documents
LLMs like OpenAI’s ChatGPT and Anthropic’s Claude transformed how finance teams retrieve insights, document processes, and generate analysis, using everyday language instead of technical queries.
The ability to interpret and produce context-aware text gave finance professionals unprecedented access to information and automation. However, limitations in accuracy, hallucinations, and lack of autonomy highlighted the need for more reliable and controllable systems.
Fifth Generation: Agentic AI (Present)
Agentic AI represents the most advanced stage in the evolution of artificial intelligence in finance. Unlike previous generations that respond to prompts or follow static models, agentic systems can perceive, reason, and act independently.
They proactively identify tasks, make decisions, learn from outcomes, and collaborate with human users to drive results, while maintaining transparency and control.
Real-World Applications:
- Autonomous financial planning and forecasting
- Proactive identification and resolution of data discrepancies
- Adaptive compliance monitoring and audit preparation
- Self-directed month-end close coordination
- Intelligent task management across finance workflows
What sets this generation apart is its capacity for autonomy and adaptability. Agentic AI systems not only execute tasks but also determine which tasks to perform and how to approach them based on changing conditions.
As highlighted by the World Economic Forum, these models go beyond generative AI by enabling finance teams to scale judgment, not just execution—reshaping roles, processes, and expectations across the office of the CFO.
“Unlike today’s GenAI models, which respond to specific human prompts, Agentic AI can independently perceive, reason, act and learn, without constant human guidance. — World Economic Forum”
Why This Evolution Matters for Modern Finance Teams
The evolution of AI in finance isn’t just a story of technical progress—it’s a shift that directly impacts how finance teams operate, collaborate, and add strategic value. Each generation of AI has progressively reduced the manual burden on teams, allowing professionals to move away from transactional tasks and toward higher-level analysis, forecasting, and decision-making.
For multi-entity organizations juggling consolidations, reconciliations, and audit readiness, understanding where today’s tools sit on this timeline helps clarify their capabilities and limitations.
Teams still relying on spreadsheet-based or static automation systems may be stuck with inefficiencies that newer AI models are already solving elsewhere.
This historical perspective helps finance leaders evaluate not just what AI can do, but how far their current tools can scale. As complexity increases, so does the need for intelligent systems that can adapt, learn, and proactively surface insights without waiting on human intervention.
Looking Ahead
The evolution of artificial intelligence in finance has moved far beyond automation: it now powers systems that can learn, adapt, and act with autonomy.
As technology continues to advance, the focus will shift toward building intelligent, transparent systems that can manage complexity without compromising compliance or control.
For finance and accounting professionals, understanding this evolution isn’t optional; it’s essential. It provides the context to evaluate modern tools, identify what’s holding teams back, and prepare for what’s coming next.
AI won’t replace human expertise, but it will redefine how that expertise is applied, and elevate the teams who embrace it.
One of the clearest ways this transformation is already playing out is in financial consolidation, where AI-driven systems are replacing spreadsheets and manual eliminations with speed, accuracy, and real-time insights. If your team is still navigating close cycles the old way, now is the time to rethink what’s possible.
See how consolidation is evolving in parallel with AI: The Evolution of Financial Consolidation.